Audible curates personalized recommendations for users by analyzing their listening habits and preferences. Through a combination of algorithms and user data, audible tailors its suggestions to match individual interests and tastes, enhancing the user experience.
These personalized recommendations help users discover new audiobooks that align with their preferences, ultimately increasing user engagement and satisfaction. By leveraging advanced technology and understanding user behavior, audible delivers a unique and personalized experience to each individual listener. Whether it’s suggesting books from similar genres, recommending popular titles, or highlighting personalized collections, audible ensures that users always have access to content they will enjoy.
So, let’s explore how audible curates personalized recommendations for users in more detail.
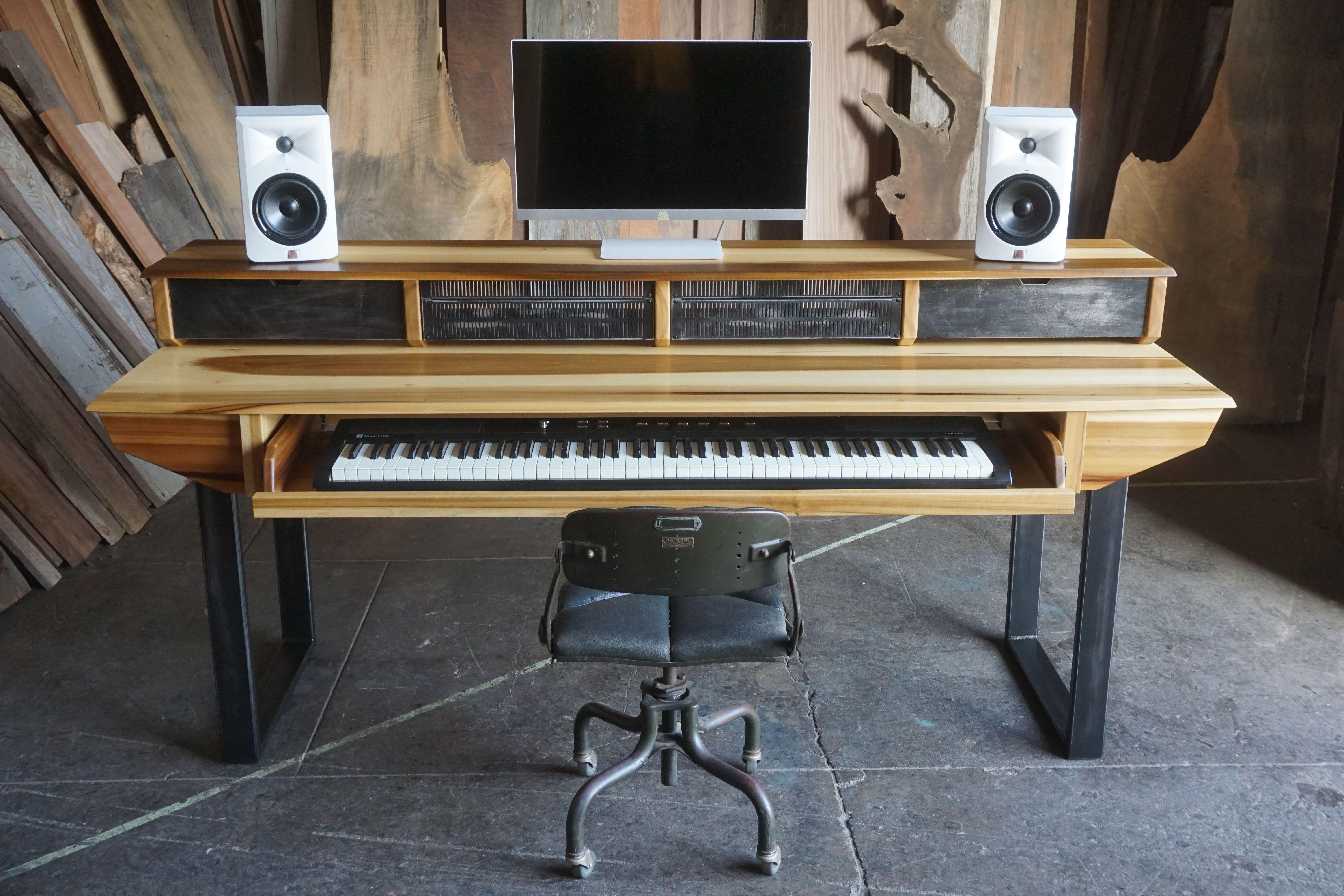
Credit: www.custommade.com
Understanding User Preferences
When it comes to curating personalized recommendations, audible takes into account various factors to understand user preferences. By analyzing user listening history, gathering insights from ratings and reviews, and considering other key factors, audible is able to provide tailored recommendations that cater to individual tastes and interests.
Let’s dive into the specific factors that influence how audible curates personalized recommendations for users.
Factors Influencing Personalized Recommendations
Audible considers several factors to deliver personalized recommendations that align with user preferences. These factors include:
- Analyzing user listening history: Audible carefully examines the types of audiobooks users have consumed in the past. By analyzing the genres, authors, and specific titles that users have engaged with, audible gains valuable insights into their preferences and can recommend similar content that suits their tastes.
- Gathering insights from user ratings and reviews: Audible takes user feedback seriously. By analyzing ratings and reviews, audible gains a deeper understanding of what users enjoy and dislike about specific audiobooks. This valuable feedback helps audible fine-tune its recommendations to better match users’ preferences.
- Considering user interests and habits: In addition to listening history, audible considers other user interests and habits. This may include factors such as preferred genres, authors, narrators, and even listening patterns (such as duration and preferred times of day). By taking these into account, audible can offer personalized recommendations that resonate with users on a deeper level.
- Incorporating browsing behavior: Audible pays attention to users’ browsing behavior on the platform. By analyzing which books users frequently search for, add to their wishlists, or explore further, audible can better understand their specific interests and provide relevant recommendations accordingly.
- Factoring in community trends: Audible also considers broader trends within its user community. By analyzing which audiobooks are trending amongst similar user profiles, audible can identify patterns and recommend popular titles that may appeal to a wider audience with similar tastes.
- Utilizing machine learning algorithms: Audible’s recommendation system utilizes sophisticated machine learning algorithms that continuously learn from user interactions and data. These algorithms take into account the aforementioned factors to generate personalized recommendations that improve over time.
By considering user listening history, gathering insights from ratings and reviews, and factoring in various preferences and behaviors, audible is able to deliver highly personalized recommendations that cater to individual tastes. Whether users are seeking thrilling mysteries, educational non-fiction, or captivating biographies, audible strives to provide a tailored and enjoyable listening experience for each and every user.
Machine Learning Algorithms
Machine learning algorithms play a crucial role in curating personalized recommendations for users on audible. By analyzing user behavior and preferences, these algorithms can understand individual tastes and deliver tailored suggestions. Here are the key points to note:
- Collaborative filtering is one of the machine learning techniques used by audible. It compares a user’s interests with other users who have similar tastes and recommends books that those like-minded individuals enjoyed. This approach leverages collective wisdom to identify hidden patterns and make accurate suggestions.
- Content-based filtering is another technique employed by audible’s recommendation system. This method focuses on the characteristics of the books themselves, rather than relying solely on user behavior. It analyzes attributes such as genre, author, narrator, and themes to recommend books that align with a user’s specific preferences.
- Hybrid recommendation systems combine the strengths of collaborative and content-based filtering to provide even more personalized suggestions. By incorporating both user behavior and book attributes, audible can create a comprehensive understanding of a user’s preferences and interests. This approach ensures a more accurate and diverse range of book recommendations.
- Thanks to machine learning algorithms, audible can continuously adapt and improve its recommendations. These algorithms learn from user feedback and adjust the suggestions accordingly to enhance the overall user experience.
Utilizing Collaborative Filtering:
- Audible uses collaborative filtering to suggest books based on similarities between a user and other like-minded individuals.
- This technique taps into collective behavior to uncover hidden patterns and make pinpointed recommendations.
- By analyzing the preferences of similar users, audible can suggest books that the user may have otherwise missed.
Implementing Content-Based Filtering:
- Audible’s recommendation system also utilizes content-based filtering.
- Rather than solely relying on user behavior, this approach analyzes book attributes such as genre, author, narrator, and themes.
- By understanding a user’s preferences based on these characteristics, audible can suggest books that align with their specific interests.
Incorporating Hybrid Recommendation Systems:
- Audible combines collaborative and content-based filtering in its recommendation systems.
- By utilizing both approaches, audible ensures a more comprehensive understanding of a user’s preferences and interests.
- This hybrid approach provides a more accurate and diverse range of book recommendations.
- With continuous learning and adaptation, these hybrid systems help audible improve its recommendations over time.
Machine learning algorithms are the driving force behind audible’s personalized recommendation system. Through collaborative filtering, content-based filtering, and hybrid approaches, audible delivers tailored book suggestions that match each user’s unique tastes and preferences. As these algorithms continue to learn and adapt, users can expect even more precise and enjoyable recommendations.
Data Analytics And User Segmentation
Utilizing Big Data Analytics For User Segmentation
In order to curate personalized recommendations for users, audible utilizes big data analytics to segment its users effectively. This allows audible to understand each user’s listening habits, preferences, and interests on a granular level. By leveraging the power of data, audible can provide a tailored experience to each individual user, enhancing their overall satisfaction with the platform.
Here are key points on how audible utilizes big data analytics for user segmentation:
- Analyzing vast amounts of data: Audible collects and analyzes vast amounts of data from its users, including listening history, user ratings, browsing behavior, and more. This data is processed and used to identify patterns and trends among different user segments.
- Identifying user behavior patterns: Through sophisticated data analysis techniques, audible is able to identify patterns and trends in user behavior. This includes the types of books users listen to, the duration of their listening sessions, their preferred genres, and more.
- Creating user profiles: Based on the analysis of user behavior, audible creates individual user profiles. These profiles capture the unique preferences and interests of each user, allowing for personalized recommendations to be generated.
- Machine learning algorithms: Audible leverages machine learning algorithms to analyze user data and refine its understanding of each user’s preferences. These algorithms continuously learn and adapt based on user interactions, improving the accuracy of personalized recommendations over time.
- Fine-grained segmentation: Using data analytics, audible is able to segment users into fine-grained groups based on various factors such as genre preferences, listening frequency, and favorite authors. This allows for highly targeted recommendations that align with each user’s specific interests.
By utilizing big data analytics for user segmentation, audible can provide an enhanced listening experience for its users. Through data-driven insights and personalized recommendations, audible ensures that users discover new and relevant audiobooks that align with their individual interests and preferences.
The Role Of Natural Language Processing
Using Nlp To Understand User Preferences
Natural language processing (nlp) plays a crucial role in curating personalized recommendations on audible. By analyzing user interactions and book summaries, audible harnesses the power of nlp to gain insights into users’ preferences. Here’s how it works:
- Nlp helps audible understand the unique preferences of each user by analyzing their interactions with the platform. It takes into account factors like browsing history, listening habits, and user input to create a detailed profile of their interests.
- By processing book summaries, nlp identifies key themes, genres, and topics within the content. It extracts relevant information that can be used to suggest books tailored to the user’s specific tastes.
- The powerful algorithms behind audible’s nlp capabilities go beyond surface-level analysis. They delve deep into the content and extract semantic meaning to truly understand the essence of each book.
- With nlp’s ability to comprehend the subtleties of language, audible can identify similarities between books and draw connections that may not be immediately apparent. This leads to more accurate and relevant recommendations.
- Nlp empowers audible to adapt to changing user preferences over time. As users engage with different content, the algorithms learn and adjust, ensuring that recommendations remain up to date.
Analyzing Book Summaries For Relevant Recommendations
Book summaries are a treasure trove of valuable information, and audible utilizes nlp to analyze them effectively. Here’s how the analysis of book summaries helps audible curate tailored recommendations:
- Nlp algorithms parse through book summaries to identify keywords and phrases that encapsulate the essence of each book. This process helps establish the genre, theme, and tone of the content.
- By understanding the key elements of each book, audible can offer recommendations that align with the user’s preferences. Whether someone enjoys thrillers, biographies, or fantasy novels, analyzing the summaries allows audible to suggest the most relevant titles.
- Book summaries provide concise overviews, but nlp takes it a step further by diving deep into the content itself. By analyzing the language and context, audible’s algorithms gain a comprehensive understanding of what makes each book unique.
- Nlp-based analysis also considers user feedback and reviews, helping audible take into account the opinions of others who have consumed the same content. This collective intelligence enriches the recommendations and ensures a personalized touch.
Extracting Keywords And Themes From User Interactions
Audible goes beyond book summaries and delves into user interactions to extract keywords and themes. Let’s explore how this process strengthens the personalized recommendations:
- Nlp algorithms analyze user input, such as searches, book ratings, and reviews, to understand their preferences. By identifying patterns and extracting keywords, audible gains insights into the topics that resonate with each user.
- Understanding user interactions is crucial for audible to deliver accurate recommendations. By leveraging nlp, audible can identify the specific themes that capture a user’s interest, be it historical fiction, self-help, or science fiction.
- The combination of book summaries and user interactions allows audible to create a comprehensive profile for each user. This profile is continuously updated and refined, ensuring that recommendations evolve alongside the user’s changing preferences.
- With the help of meaningful data extracted from user interactions, audible’s nlp-powered algorithms can detect subtle nuances in preferences. This makes the recommendations more precise, enhancing the overall listening experience.
By leveraging the power of natural language processing, audible delivers highly personalized recommendations that cater to the unique preferences of each user. The analysis of book summaries and user interactions allows audible to curate a vast selection of books tailored to the individual’s tastes, making the listening experience truly exceptional.
Incorporating Social Network Analysis
Harnessing Social Connections For Recommendations
Incorporating social network analysis into its recommendation algorithm allows audible to curate personalized recommendations for its users. By leveraging user interactions and networks, audible can tap into the power of social connections to provide tailored book suggestions. Here’s how they do it:
- Audible analyzes the social connections of its users, including friends, family, and acquaintances, to identify potential book recommendations.
- The algorithm considers factors such as mutual connections, similar reading preferences, and listening habits to determine the relevance and suitability of a book recommendation.
- Through social network analysis, audible identifies influential users who have a significant impact on others’ reading choices. These users’ recommendations carry more weight in the personalized suggestions.
Leveraging User Interactions And Networks
Audible understands that reading is often a social activity, influenced by our connections with others. By incorporating user interactions and networks into its recommendation process, audible creates a more engaging and personalized experience. Here’s how it works:
- Audible tracks user interactions within the platform, including book ratings, reviews, and recommendations.
- User interactions provide valuable data that helps audible understand the individual’s interests, preferences, and reading habits.
- Audible takes into account the books that a user’s friends or connections have experienced and enjoyed. If multiple connections have similar preferences, audible factors in those recommendations when tailoring suggestions for the user.
Audible’s approach to personalized recommendations goes beyond individual preferences. By harnessing social connections and leveraging user interactions and networks, audible ensures that users receive book suggestions that align with their interests while also considering the influence of their connections. This combination of social network analysis and user interactions creates a more impactful and engaging recommendation system for audible users.
Personalized Recommendations On Audible’S Home Page
Customizing the home page based on user preferences:
- Audible curates personalized recommendations on its home page to enhance the user experience.
- The home page is tailored to showcase content that aligns with the user’s preferences and interests.
- Algorithms analyze the user’s listening history, ratings, reviews, and browsing behavior to create a customized experience.
- By understanding individual preferences, audible can deliver books and genres that are likely to resonate with each user.
- This personalization allows users to easily discover new titles they might enjoy, saving time and effort.
Showcasing recommended books in various categories:
- Audible’s home page features recommended books across different categories, ensuring a diverse selection.
- Categories include fiction, non-fiction, mystery, romance, self-help, fantasy, and many more.
- Users can easily navigate through different genres and find books that appeal to their specific interests.
- Audible’s extensive library enables users to explore new authors, bestsellers, and hidden gems.
- The variety of categories helps cater to different tastes and encourages users to step out of their reading comfort zones.
Fine-tuning recommendations for individual users:
- Audible goes beyond broad categories to fine-tune recommendations for each user.
- The algorithm takes into account numerous factors, such as past purchases, wishlist items, and listening habits.
- By analyzing this data, audible can understand an individual’s preferences on a granular level.
- The algorithm adapts over time, learning from user interactions and continuously refining recommendations.
- This personalized approach ensures that users receive suggestions that closely align with their unique tastes and preferences.
Audible’s personalized recommendations on the home page offer users a tailored experience. By customizing the content based on user preferences, showcasing recommendations in various categories, and fine-tuning recommendations for individual users, audible helps users discover books they are likely to enjoy and expands their reading horizons.
Feedback Loop And Continuous Learning
Incorporating user feedback for improved recommendations:
- Audible understands that feedback from users is invaluable in enhancing their recommendation algorithms. By actively seeking and analyzing user feedback, audible can gain insights into individual preferences and interests, allowing them to tailor recommendations more accurately.
- Users can provide feedback through various means, such as rating audiobooks, leaving reviews, or indicating their listening preferences in their profiles. Audible pays close attention to this feedback, using it as a crucial input for improving their algorithms and recommendation systems.
- Through these feedback loops, audible can identify patterns and trends in user preferences and adjust their algorithms accordingly. This iterative process helps to deliver more personalized and relevant recommendations to users over time.
Iteratively updating and refining recommendation algorithms:
- Audible employs a continuous learning approach to refine its recommendation algorithms. By analyzing user data on an ongoing basis, audible can identify patterns and trends that help improve their recommendations.
- Through machine learning techniques, audible can evaluate the listening habits and preferences of millions of users. This data is then used to update and refine the recommendation algorithms, ensuring that users receive tailored suggestions that align with their interests.
- Audible’s algorithms take into account various factors, such as genre preferences, past listening history, user ratings, and the recommendations of similar users. This iterative process allows audible to continually fine-tune their algorithms, enhancing the accuracy and relevance of their recommendations.
Enhancing the user experience through data-driven insights:
- The vast amount of user data that audible collects allows them to gain valuable insights into user behavior and preferences. These insights are then leveraged to enhance the overall user experience.
- By analyzing user data, audible can identify listening patterns, genre preferences, and emerging trends within the audiobook industry. This information enables them to make data-driven decisions that optimize the user experience.
- Data-driven insights also help audible identify gaps in their content library and prioritize the acquisition of new audiobooks to meet user demand. This ensures a diverse and extensive range of recommendations that cater to different tastes and interests.
Audible’s personalized recommendation system utilizes a feedback loop and continuous learning approach to enhance the user experience. By incorporating user feedback, updating and refining algorithms iteratively, and drawing insights from data, audible strives to provide highly personalized and relevant recommendations to its users.
Privacy And Data Security
Audible is renowned for its personalized recommendations that keep users coming back for more. But how exactly does audible curate these recommendations? In this section, we’ll explore the measures taken by audible to safeguard user data and privacy, as well as its commitment to complying with data protection regulations and ensuring transparency in data usage.
Safeguarding User Data And Privacy
- Audible recognizes the importance of protecting user data and privacy as a top priority.
- Industry-leading security measures are implemented to safeguard personal information.
- Stringent access controls are in place to restrict unauthorized access to user data.
- Advanced encryption techniques are employed to protect sensitive information.
- Regular security audits and vulnerability assessments are conducted to identify and address potential risks.
Complying With Data Protection Regulations
- Audible fully complies with data protection regulations such as the general data protection regulation (gdpr).
- User data is collected and processed strictly in accordance with the applicable laws and regulations.
- Audible respects users’ rights regarding their personal data, including the right to access, rectify, and delete their information.
- Transparent opt-in and opt-out mechanisms are provided to give users control over their data.
Ensuring Transparency In Data Usage
- Audible maintains a transparent approach towards the usage of user data.
- Clear privacy policies and terms of service are readily available for users to review.
- Users are informed about the types of data collected and how it is used to personalize recommendations.
- Audible ensures that user data is used solely for the purpose of enhancing the user experience.
- Any sharing of data with third parties is done with explicit user consent and only when necessary for service provision.
By prioritizing privacy and data security, audible demonstrates its commitment to protecting user information while delivering personalized recommendations.
Conclusion
Audible takes a multi-faceted approach to curate personalized recommendations for its users. By leveraging advanced algorithms and machine learning techniques, as well as incorporating user behavior data and preferences, audible is able to provide tailored book recommendations. The use of collaborative filtering and content-based filtering methods ensures that users are presented with books that align with their interests and reading habits.
Additionally, audible’s integration with amazon allows for further personalization, as it takes into account users’ purchase history and reviews. The incorporation of human curation further enhances the recommendation process, ensuring that the suggestions are of high quality and relevance. Audible’s goal is to continuously refine and improve its recommendation system, delivering a highly personalized and engaging user experience.
So whether you’re a fan of mysteries, romance, or non-fiction, audible has got you covered with its personalized book recommendations. Discover your next great listen today!